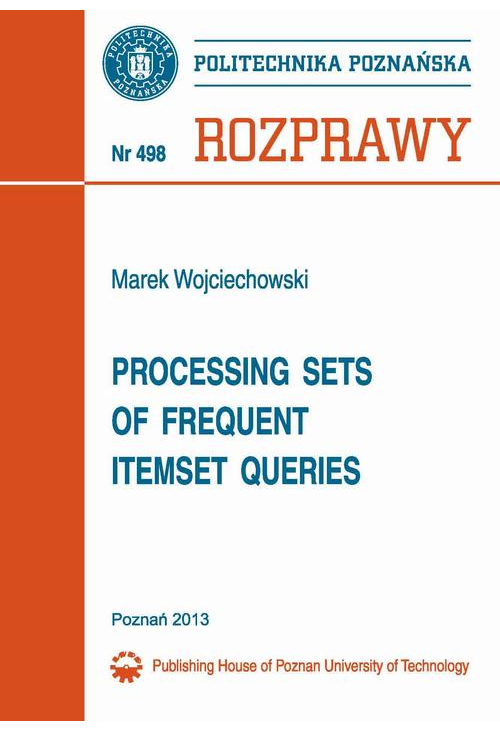
Ebook Processing sets of frequent itemset queries
Opis treści
This dissertation is devoted to frequent itemset mining regarded as advanced database querying where users specify the source dataset, the minimum frequency threshold, and optionally pattern constraints narrowing the results, and it is up to the data mining system to execute the mining task as efficiently as possible. Building upon existing solutions optimizing the execution of individual queries or sequences of queries, we bring frequent itemset query optimization to another level and consider the problem of efficient processing of sets of frequent itemset queries, analogous to multi-query optimization in database systems. Our solutions target mainly batch processing mode but can be applied to multi-user interactive environments as well.In this dissertation we formulate the problem of processing sets of frequent itemset queries in the context of a simple, general model of frequent itemset queries independent of particular languages and interfaces, and provide several solutions addressing the problem. The majority of the developed techniques are defined in terms of a data sharing model based on the concept of elementary data selection predicates which represent parts of the dataset shared among the queries. The developed methods of processing sets of frequent itemset queries can be broadly classified into two categories: methods independent of a particular frequent itemset mining algorithm, and the ones designed with a specific algorithm in mind. The explicitly addressed frequent itemset mining algorithms are: Apriori, FP-growth, and Partition, which we claim belong to the most influential ones, and in addition are important from the point of view of possible practical applications. All the proposed techniques are initially formulated and experimentally verified under the assumption that data partitions corresponding to elementary data selection predicates can be selectively retrieved from the database. Afterwards, theoretical and experimental analysis of the influence of available access paths to data on the proposed techniques is conducted.
An important contribution of the dissertation is related to the identified optimization problem occurring in one of the techniques for the Apriori algorithm. The problem concerns handling large batches of queries by dividing the set of queries into subsets executed independently. For the problem formulated as a particular case of hypergraph partitioning, its NP-hardness is proved and several heuristic solutions are provided.
Rozprawa jest poświęcona problemowi odkrywania zbiorów częstych poprzez tzw. zapytania eksploracyjne stanowiące specyfikację zbioru danych źródłowych, wymaganej minimalnej częstości występowania oraz opcjonalnie ograniczeń nakładanych na odkrywane wzorce. Opierając się na istniejących rozwiązaniach w zakresie optymalizacji wykonania pojedynczych zapytań eksploracyjnych oraz sekwencji takich zapytań,
w rozprawie przeniesiono optymalizację zapytań eksploracyjnych dotyczących problemu odkrywania zbiorów częstych na nowy poziom, koncentrując się na optymalizacji wykonania zbiorów zapytań eksploracyjnych, stanowiącej koncepcyjne nawiązanie do optymalizacji zbiorów zapytań w systemach baz danych. Proponowane rozwiązania odnoszą się głównie do systemów eksploracji danych przetwarzających zadania w trybie wsadowym, ale mogą znaleźć zastosowanie również w systemach wielodostępnych, obsługujących wiele współbieżnych sesji interaktywnych.
W rozprawie sformułowano problem przetwarzania zbiorów zapytań eksploracyjnych w kontekście prostego, ogólnego modelu zapytań dotyczącego problemu odkrywania zbiorów częstych, niezależnego od konkretnych języków oraz interfejsów wykorzystywanych w eksploracji danych, i zaproponowano szereg rozwiązań postawionego problemu. Większość opracowanych technik odnosi się do zaproponowanego modelu współdzielenia danych przez zapytania eksploracyjne, opartego na rozłącznych formułach selekcji reprezentujących podzbiory zbioru danych współdzielone przez zapytania. Przedstawione w rozprawie metody przetwarzania zbiorów zapytań eksploracyjnych dotyczących problemu odkrywania zbiorów częstych można ogólnie podzielić na dwie kategorie: metody niezależne od konkretnego algorytmu odkrywania zbiorów częstych oraz te zaprojektowane z myślą o konkretnym algorytmie. Specyficzne metody wykonania zbiorów zapytań zostały opracowane dla algorytmów Apriori, FP-growth i Partition, które należą do najbardziej znaczących algorytmów odkrywania zbiorów częstych i jednocześnie są istotne z punktu widzenia potencjalnych zastosowań. Wszystkie zaproponowane techniki zostały najpierw sformułowane i eksperymentalnie zweryfikowane przy założeniu, że partycje danych odpowiadające rozłącznym formułom selekcji mogą być selektywnie odczytane z bazy danych. Następnie przeprowadzono teoretyczną i eksperymentalną analizę wpływu ścieżek dostępu do danych na ich wydajność.
Ważnym elementem rozprawy jest zidentyfikowany problem optymalizacyjny, występujący w jednej z technik zaproponowanych dla algorytmu Apriori. Problem ten dotyczy obsługi dużych zbiorów zapytań eksploracyjnych poprzez ich podział na niezależnie wykonywane podzbiory. Problem został sformułowany jako szczególny przypadek partycjonowania hipergrafu, udowodniono jego NP-trudność, a także opracowano dla niego kilka heurystyk.
Spis treści ebooka Processing sets of frequent itemset queries
Abstract 6
1. Introduction 7
1.1. Data Mining from a Database Perspective 7
1.2. Aim and Scope of the Dissertation 11
2. Frequent Itemset Mining 14
2.1. Overview, Genesis, Applications, and Importance of the Problem 14
2.2. Formulation of the Frequent Itemset Mining Problem 16
2.3. Computational Complexity of the Problem 18
2.4. Overview of Approaches to Frequent Itemset Mining 19
2.4.1. Introduction 19
2.4.2. Search Space Traversal Strategies 20
2.4.3. Database Layout 23
2.4.4. Using Memory to Store Mined Data 26
2.4.5. Itemset Support Counting 27
2.5. Representative Frequent Itemset Mining Algorithms 28
2.5.1. Introduction 28
2.5.2. Apriori 30
2.5.3. FP-growth 36
2.5.4. Partition 39
2.6. Research Trends in Frequent Itemset Mining 41
2.6.1. Introduction 41
2.6.2. Taking Advantage of DBMS Functionality in Frequent Itemset Mining 42
2.6.3. Sampling for Frequent Itemset Mining 44
2.6.4. Concise Representations of Frequent Itemsets 46
2.6.5. Parallel and Distributed Frequent Itemset Mining 49
2.6.6. Frequent Itemset Mining over Data Streams 52
2.6.7. Privacy Preserving Frequent Itemset Mining 54
3. Data Mining as Advanced Querying 57
3.1. Motivation 57
3.2. Prototype Data Mining Query Languages 57
3.3. Data Mining Standards 60
3.4. Data Mining Queries in Contemporary Database Management Systems 67
3.5. Data Mining Queries: Summary of the Current State of the Art and Implications 71
4. Frequent Itemset Query Processing 73
4.1. Constraint-based Frequent Itemset Mining 73
4.2. Reusing Results of Frequent Itemset Queries 76
4.3. Reusing Results vs. Pushing Constraints into the Mining Process 80
5. Processing Batches of Frequent Itemset Queries 82
5.1. Motivation 82
5.2. General Model of Frequent Itemset Queries 83
5.3. Batches of Frequent Itemset Queries and Problem Formulation 85
5.4. Model of Query Data Sharing 87
5.5. Related Work 90
6. Methods Independent of the Mining Algorithm 92
6.1. Sequential Processing with Result Caching and Reusing 92
6.2. Result Filtering and Incremental Mining 93
6.3. Query Scheduling 97
6.4. Query Scheduling with Intermediate Queries 100
6.5. Mine Merge 106
6.6. Experimental Results 111
6.7. Summary and Discussion 116
7. Methods for the Apriori Algorithm 118
7.1. Common Counting 118
7.2. Common Counting with Query Partitioning 120
7.2.1. Motivation 120
7.2.2. Key Issues 121
7.2.3. Query Partitioning as a Case of Hypergraph Partitioning 124
7.2.4. Computational Complexity of the Problem 128
7.2.5. Algorithm CCRecursive 131
7.2.6. Algorithm CCFull 133
7.2.7. Algorithm CCCoarsening 136
7.2.8. Algorithm CCAgglomerative 139
7.2.9. Algorithm CCAgglomerativeNoise 140
7.2.10. Algorithm CCGreedy 142
7.2.11. Algorithm CCSemiGreedy 144
7.3. Common Candidate Tree 145
7.4. Experimental Results 148
7.4.1. Query Partitioning for Common Counting 148
7.4.2. Common Counting vs. Common Candidate Tree 159
7.5. Summary and Discussion 169
8. Methods for the FP-growth Algorithm 171
8.1. Common Building 171
8.2. Common FP-tree 173
8.3. Experimental Results 176
8.4. Summary and Discussion 182
9. Methods for the Partition Algorithm 186
9.1. Integration of Dataset Scans for Partition 186
9.2. Partition Mine Merge Improved 187
9.3. Experimental Results 191
9.4. Summary and Discussion 195
10. Data Access Methods in Processing Sets of Frequent Itemset Queries 197
10.1. Comparison of Proposed Techniques in Terms of Data Access Schemes 197
10.2. Data Organization and Access Methods in Contemporary DBMSs 199
10.3. Techniques of Processing Sets of Frequent Itemset Queries with Full Table Scans 202
10.4. Theoretical Cost Analysis 204
10.5. Experimental Results 207
10.6. Summary and Discussion 214
11. Conclusions and Future Work 216
Bibliography 221
Streszczenie 238
Szczegóły ebooka Processing sets of frequent itemset queries
- Wydawca:
- Wydawnictwo Politechniki Poznańskiej
- Rok wydania:
- 2013
- Typ publikacji:
- Ebook
- Język:
- angielski
- Format:
- ISBN:
- 978-83-7775-265-4
- ISBN wersji papierowej:
- 978-83-7775-265-4
- Wydanie:
- 1
- Autorzy:
- Marek Wojciechowski
- Miejsce wydania:
- Poznań
- Liczba Stron:
- 240
- Czas realizacji zamówienia:
- Do 10 min
@CUSTOMER_NAME@
@COMMENT_TITLE@
@COMMENT_COMMENT@